Entry Point AI
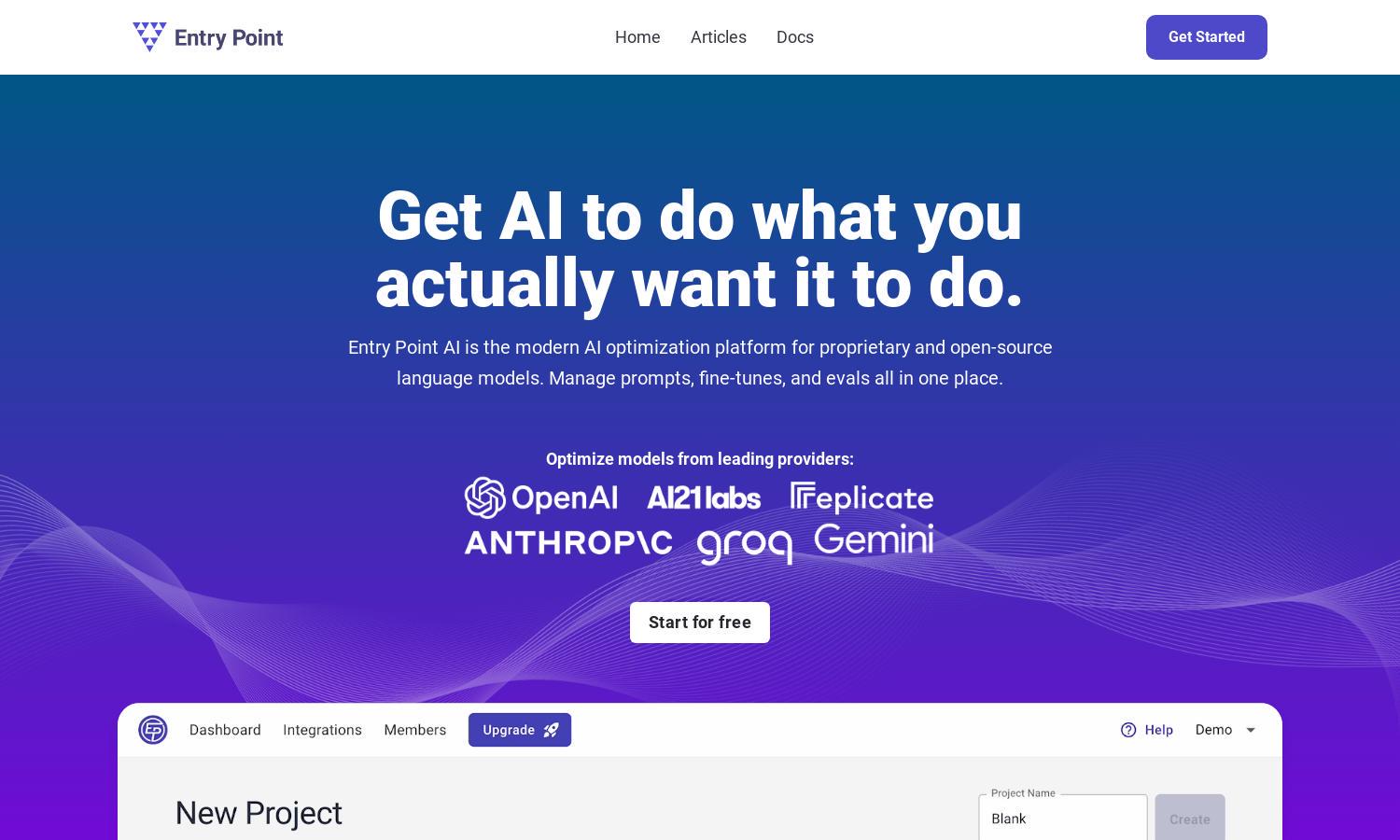
About Entry Point AI
Entry Point AI is a modern platform for fine-tuning large language models, aimed at businesses and developers. Its innovative no-code approach simplifies training, management, and evaluation of LLMs, allowing users to harness AI effectively and enhance model performance without complex coding.
Entry Point AI offers a freemium model, enabling users to start for free, with options to scale as their needs grow. With various subscription tiers offering additional features, users can unlock advanced functionalities to optimize their large language models and achieve better results.
Entry Point AI features a clean, intuitive user interface designed to streamline the fine-tuning process. Its layout facilitates easy navigation, enabling users to quickly access essential tools and resources while harnessing advanced functionalities for fine-tuning models seamlessly and efficiently.
How Entry Point AI works
Users start by signing up on Entry Point AI, selecting their desired large language model, and utilizing the user-friendly interface to import their datasets. The platform guides users through the fine-tuning process with templates, allowing for easy management of prompts and examples, resulting in optimized models without the need for coding.
Key Features for Entry Point AI
No-Code Fine-Tuning
Entry Point AI's no-code fine-tuning feature allows users to effortlessly train their large language models without coding expertise. This innovative approach not only simplifies the fine-tuning process but also empowers users to achieve tailored model outputs quickly and efficiently.
Multi-Provider Support
With Entry Point AI, users enjoy the advantage of multi-provider support, enabling easy integration and switching between various large language model APIs. This flexibility helps users stay current with advancements in AI technology while optimizing their fine-tuning processes seamlessly.
Template Engine
Entry Point AI's robust template engine facilitates rapid iteration for fine-tuning datasets. By allowing users to experiment with structure and labels, it helps ensure optimal training results, making it easier to achieve the desired performance from their large language models.
You may also like:
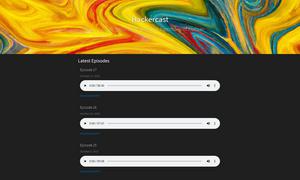